15. Functional Approximation By NN and RF#
Here we show how the function
\[
x \mapsto exp(4 x)
\]
can be easily approximated by a tree-based methods (Trees, Random Forest) and a neural network (2 Layered Neural Network)
15.1. Functional Approximation by a Tree#
install.packages("librarian", quiet = T)
librarian::shelf(rpart, randomForest, keras, gbm, quiet = T)
set.seed(1)
X_train <- matrix(runif(1000),1000,1)
Y_train <- exp(4*X_train) #Noiseless case Y=g(X)
dim(X_train)
# library(rpart)
# shallow tree
TreeModel<- rpart(Y_train~X_train, cp=.01) #cp is penalty level
pred.TM<- predict(TreeModel, newx=X_train)
plot(X_train, Y_train, type="p", pch=19, xlab="z", ylab="g(z)")
points(X_train, pred.TM, col=3, pch=19)
These packages will be installed:
'randomForest'
It may take some time.
- 1000
- 1
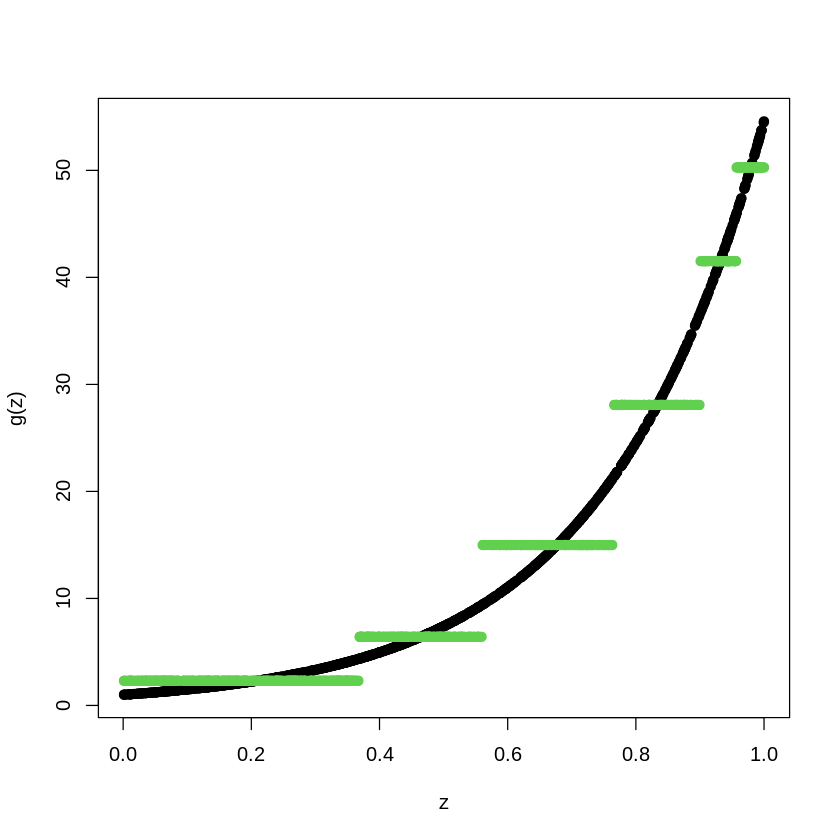
set.seed(1)
X_train <- matrix(runif(1000),1000,1)
Y_train <- exp(4*X_train) #Noiseless case Y=g(X)
dim(X_train)
# library(rpart)
TreeModel<- rpart(Y_train~X_train, cp=.0005) #cp is penalty level
pred.TM<- predict(TreeModel, newx=X_train)
plot(X_train, Y_train, type="p", pch=19, xlab="z", ylab="g(z)")
points(X_train, pred.TM, col=3, pch=19)
- 1000
- 1
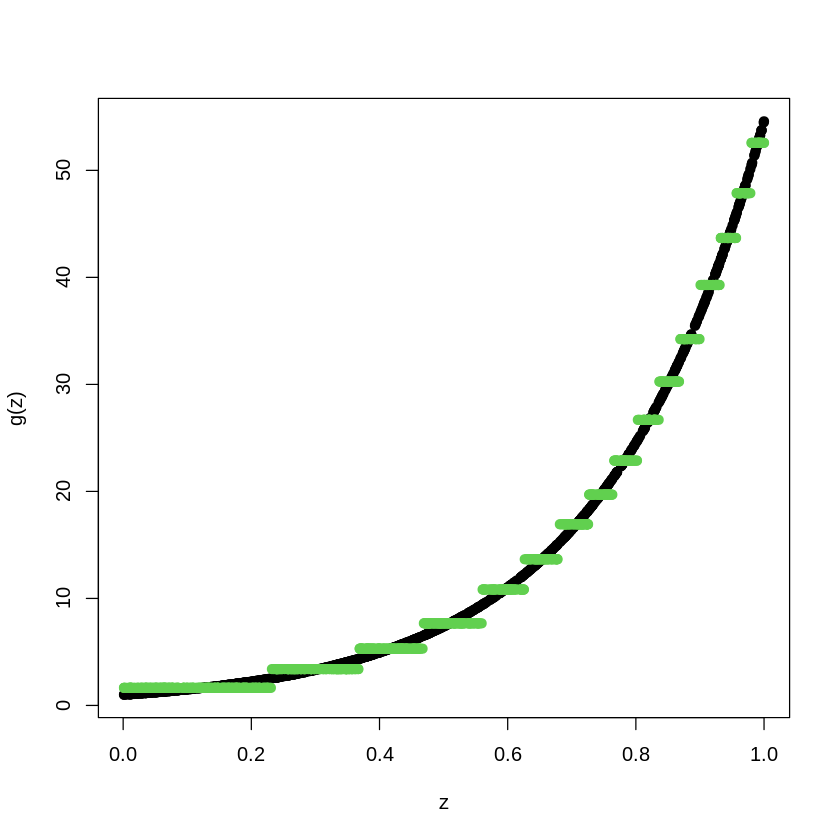
15.2. Functional Approximation by RF#
Here we show how the function
\[
x \mapsto exp(4 x)
\]
can be easily approximated by a tree-based method (Random Forest) and a neural network (2 Layered Neural Network)
# library(randomForest)
RFmodel<- randomForest(Y_train~X_train)
pred.RF<- predict(RFmodel, newdata=X_train)
plot(X_train, Y_train, type="p", pch=19, xlab="z", ylab="g(z)")
points(X_train, pred.RF, col=4, pch=19,)
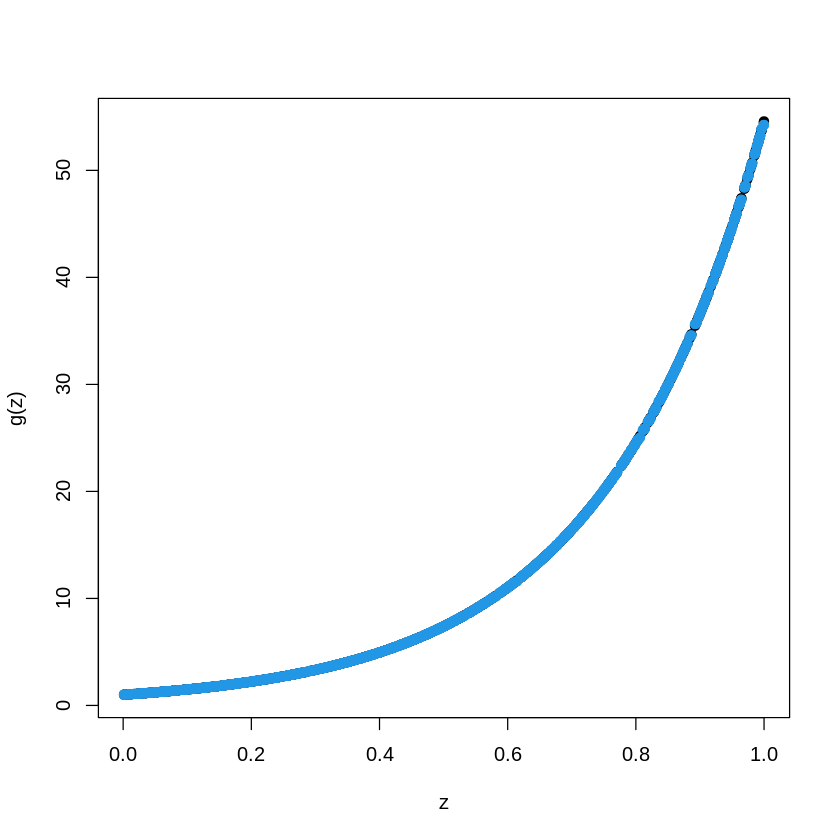
15.3. Boosted Trees#
# library(gbm)
data_train = as.data.frame(cbind(X_train, Y_train))
BoostTreemodel<- gbm(Y_train~X_train, distribution= "gaussian", n.trees=100, shrinkage=.01, interaction.depth
=4)
#shrinkage is "learning rate"
# n.trees is the number of boosting steps
pred.BT<- predict(BoostTreemodel, newdata=data_train, n.trees=100)
plot(X_train, Y_train, type="p", pch=19, xlab="z", ylab="g(z)")
points(X_train, pred.BT, col=4, pch=19,)
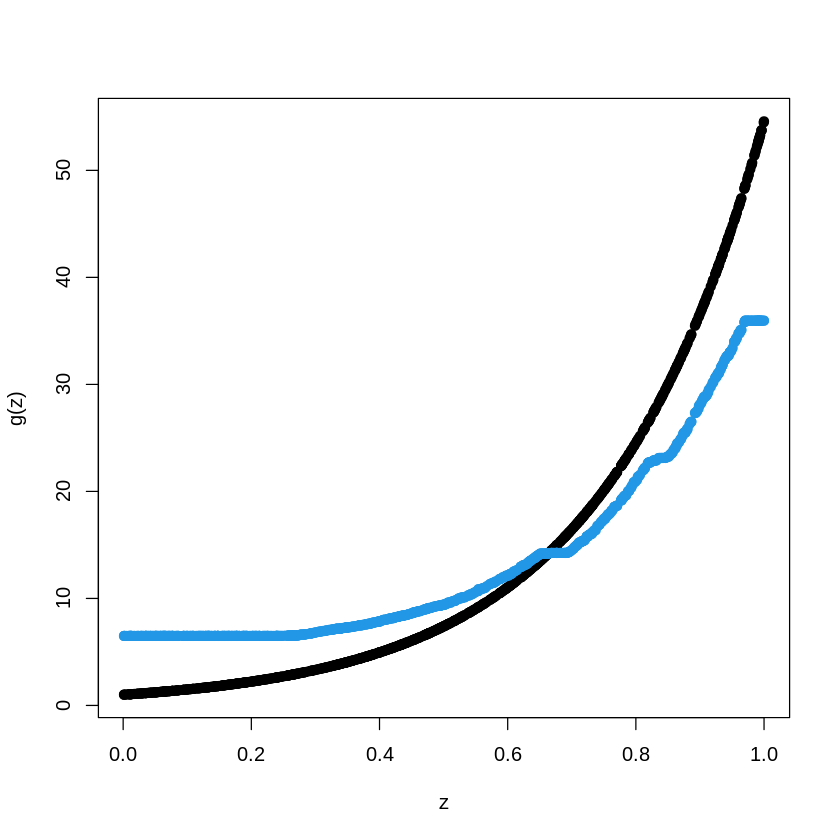
# library(gbm)
data_train = as.data.frame(cbind(X_train, Y_train))
BoostTreemodel<- gbm(Y_train~X_train, distribution= "gaussian", n.trees=1000, shrinkage=.01, interaction.depth
=4)
# shrinkage is "learning rate"
# n.trees is the number of boosting steps
pred.BT<- predict(BoostTreemodel, newdata=data_train, n.trees=1000)
plot(X_train, Y_train, type="p", pch=19, xlab="z", ylab="g(z)")
points(X_train, pred.BT, col=4, pch=19,)
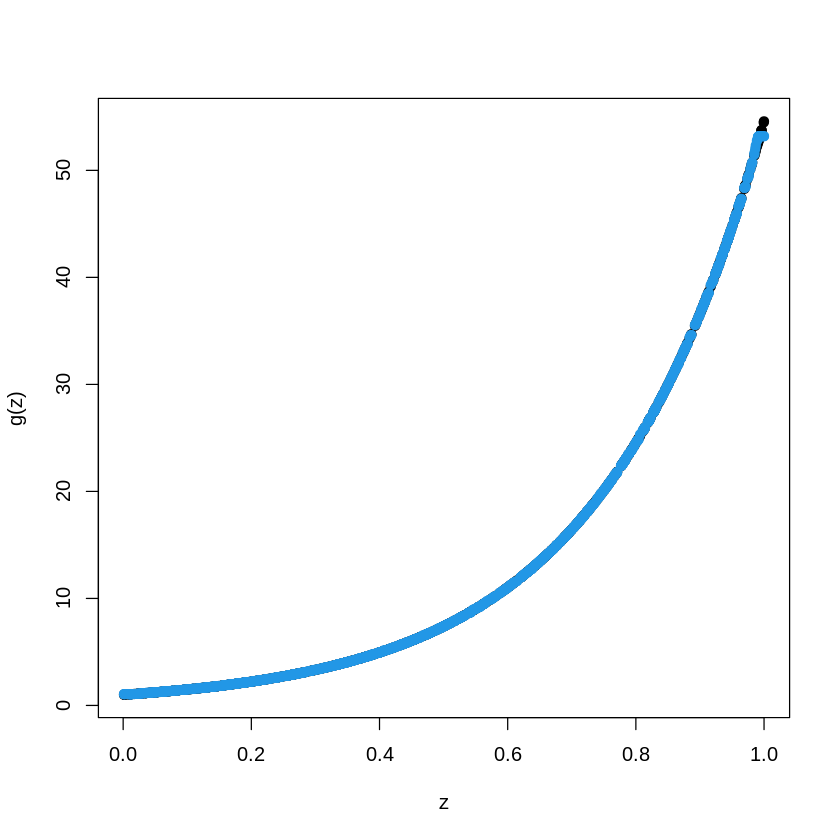
15.4. Same Example with a Neural Network#
# library(keras)
build_model <- function() {
model <- keras_model_sequential() %>%
layer_dense(units = 200, activation = "relu",
input_shape = 1)%>%
layer_dense(units = 20, activation = "relu") %>%
layer_dense(units = 1)
model %>% compile(
optimizer = optimizer_adam(lr = 0.01),
loss = "mse",
metrics = c("mae"),
)
}
model <- build_model()
summary(model)
Loaded Tensorflow version 2.8.2
Warning message in backcompat_fix_rename_lr_to_learning_rate(...):
“the `lr` argument has been renamed to `learning_rate`.”
Model: "sequential"
________________________________________________________________________________
Layer (type) Output Shape Param #
================================================================================
dense_2 (Dense) (None, 200) 400
dense_1 (Dense) (None, 20) 4020
dense (Dense) (None, 1) 21
================================================================================
Total params: 4,441
Trainable params: 4,441
Non-trainable params: 0
________________________________________________________________________________
num_epochs <- 1
model %>% fit(X_train, Y_train,
epochs = num_epochs, batch_size = 10, verbose = 0)
pred.NN <- model %>% predict(X_train)
plot(X_train, Y_train, type="p", pch=19, xlab="z", ylab="g(z)")
points(X_train, pred.NN, col=4, pch=19,)
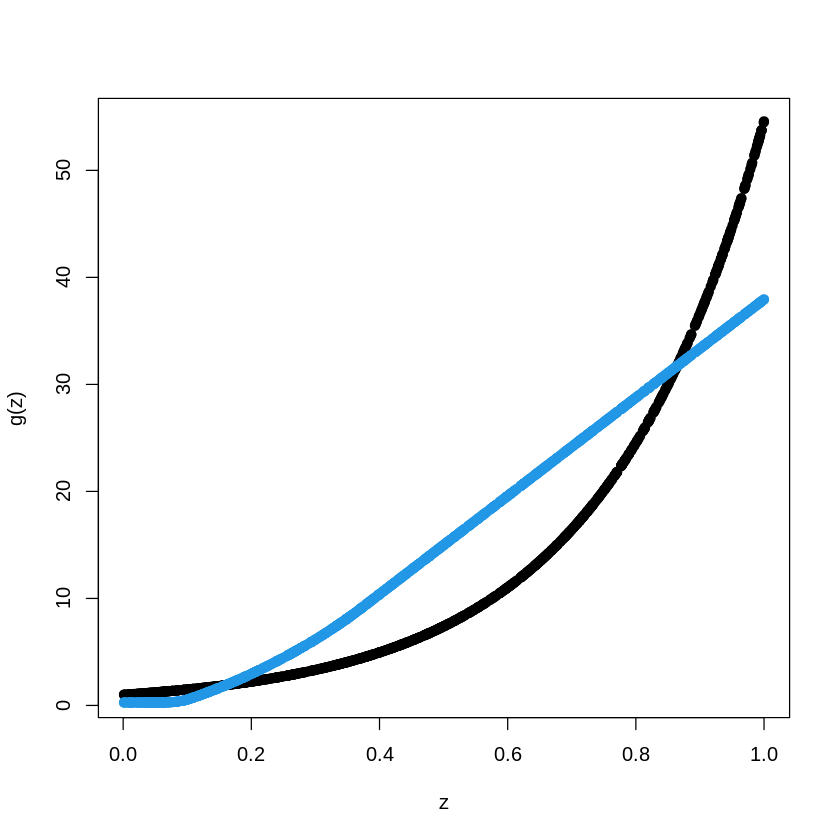
num_epochs <- 100
model %>% fit(X_train, Y_train,
epochs = num_epochs, batch_size = 10, verbose = 0)
pred.NN <- model %>% predict(X_train)
plot(X_train, Y_train, type="p", pch=19, xlab="z", ylab="g(z)")
points(X_train, pred.NN, col=4, pch=19,)
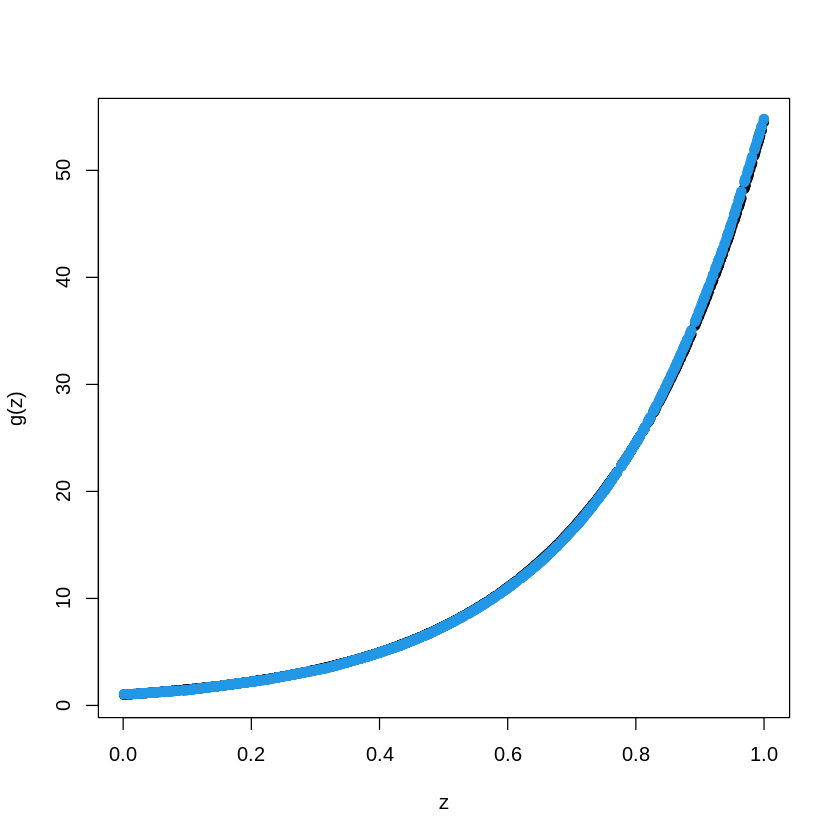